IIIT-H develops tool to count roadside trees, generate maps of urban tree cover
By Newsmeter Network Published on 29 Jun 2021 12:45 PM GMT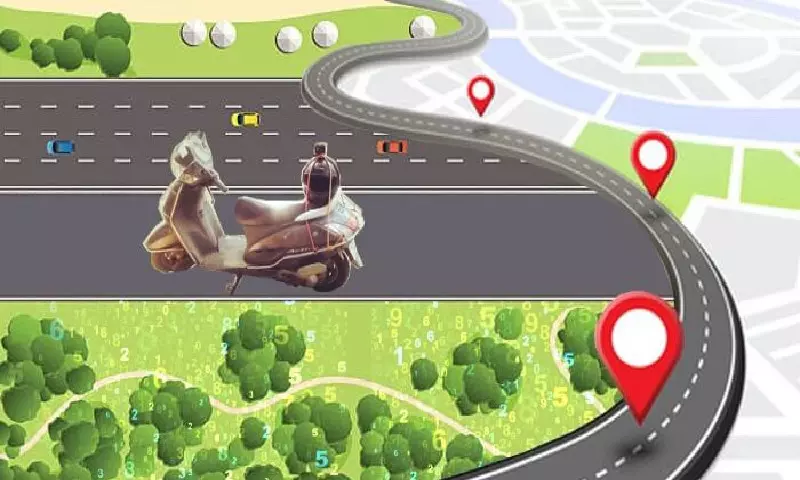
Hyderabad: In a development that could greatly assist urban planners, city councilors, and communities, the International Institute of Information Technology Hyderabad (IIITH) researchers have unveiled a computer vision-assisted tool to count roadside trees and automatically generate maps showing extent of urban tree coverage.
The importance of an urban green cover can't be stressed enough. From mitigating the effects of air and noise pollution to preventing flooding in cities by soaking up excess rainwater, the benefits of green spaces have even been linked to the health and well-being of the city population. However, it is the same greenery that is often at the receiving end of rapid urbanization.
Environmental activists and other organizations now work hand in hand with governments to make sure that for every tree axed for developmental works, an appropriate number is planted elsewhere. Or in some instances, instead of felling them, the trees are uprooted whole and replanted elsewhere. But in order to do this, one needs to assess the extent of green cover in the first place.
AI solution for future cities
Traditional methods of estimating urban canopies have involved either manually counting each tree that lies in a specified area or using satellite images to get the big picture. More recently, tree censuses have been reported with the help of unmanned aerial vehicles or drones. At the International Institute of Information Technology, Hyderabad, what started with a request from the National Institute of Urban Management to generate an AI-enabled solution to facilitate city planning and urban afforestation has now led to a computer vision-based tool.
Arpit Bahety, a research fellow at the Center for Visual Information Technology (CVIT), under the guidance of Prof. C.V Jawahar has developed a machine learning algorithm that can automatically detect and count the number of trees abutting urban roads. The system generates a colour-coded map, illustrating the extent of tree coverage on particular routes.
Colour me green
Explaining the distinction between different kinds of urban greenery such as gardens, parks, urban forests, and tree avenues, Arpit said, "For purposes of our work, we ignored bushes and shrubs and focused only on trees which typically line city streets."
With video footage of tree-lined streets obtained from Hyderabad and Delhi, the research team trained a Yolo (You only look once) v4 model – a state-of-the-art ML model for real-time object detection. It has been trained to recognize and detect trees based only on their trunks. Alongside tree detection, the algorithm also generates a tree count at regular time intervals while providing a final count at the end of a given video footage. When the tree count is fed into a GPS extraction model, the system refers to an in-house scale developed by the team where > 50 trees per km refers to a 'Very Good' tree count and < 20 represents a 'Very Low' tree count. These routes are then automatically displayed on a map with corresponding colours such as dark green for a 'Very Good' tree count and black which is the lowest on the scale.
Meeting the challenges
"Our algorithm addresses the problem of double counting (of trees) that exists in traditional methods of census taking," said Arpit while explaining how the system scores over other methods.
It was tested in Hyderabad and Surat across various city streets and the outskirts and was found to obtain an accuracy of 83%.
A visual representation of city roads with tree cover also showcases the big picture which is more useful for city planners to strategize and take appropriate steps on ways to increase tree canopy. According to Ramesh Loganathan, professor, co-innovation and outreach, IIITH, "This solution is very timely given the intense push for greening in our state, as well as in other parts. It can help quantify and track the health and growth of planted trees. Technology-wise also, this is very challenging given the wide range of plants and trees on the road, trees behind trees or inside plots, other structures that may be in front or in between, lighting conditions when capturing the videos and many more."
To overcome some limitations in tree detection algorithm especially in cases where either the trees have very thin trunks or cannot be easily identified due to objects close to them like tree guards, electricity poles, buildings and so on, the team is fine tuning the process of training the dataset. They are also refining the process of visualization via a kernel density estimation process which does not require the in-house tree density index. "It can automatically show which areas have higher tree density and which ones lower," explained Arpit.